I came accross
this book by Paul B. Brown, Charles F. Kiefer and Leonard A. Schlesinger almost by chance. The title was enticing so I decided to give it a go. You can read it really fast and the structure is very approachable. Having an Information Security mindset, you can apply generic recommendations to our professional field and even try out some entrepreneurial experiments that could lead you to a professional change.
If you are going through a period of time after which you really need a positive? work-related change, reading this book could help you. As always, a little disclaimer: This post does not replace at any time the careful reading of the book and all points expressed here are extracted from the book but by no means complete, comprehensive or unbiased.
If I would have to summarise the book in only one sentence, I would say ALBR. The acronym of Act, Learn, Build and Repeat. This is what authors recommend to put in practice your own ideas. Note that they start with the word Action. The beauty of this book comes now: You select the scope and the context in which you will apply your own ideas: in your startup, with your current employer, at home, during your leisure time... actually these learning points can be applied everywhere and anytime.
I also like a lot the fact that this book, published in 2014 also proposes something that I was already suggesting in
my first Information Security book: IT Securiteers - Information Security Management: Take baby steps, small steps so that you can always be in control and, if needed, revert back. Baby steps are an important risk-management measure.
The book is full of US-based examples. At the end of every chapter you have a nice little box with the key learning points (just as the
IT Securiteers book, where you can also find a summary of the applicable MBA models at the end of every chapter).
The first section of the book describes how our professional world has changed compared to the one previous generations had and how this fact requires new skills (and new approaches) in all of us. Worth highlighting regarding risk management, the book confirms how the best entrepreneurs are quite risk averse.
The second section actually proposes the Act/Learn/Build/Repeat process to manage risk when starting off a new endevour. This process, plus the use of small baby steps, make you ready to fail safe, since there will never be something really major, or not manageable, at stake.
The third section is very realistic. It first confirms that not all our likes and passions will be payed by the market i.e. we can only follow our passion if we can (economically and realistically) afford it. Let's remember we need to live in this world. This is a convenient time to mention the model I wrote about in the
IT Securiteers book on the intersection of your skills, your passions and the market to make a living.
The fourth section provides an interesting spin to starting something new: They propose to do it outside your everyday job. Certainly the possibility to start something new within your current job, providing even more value to your employers, should not be discarded. Actually, for those ranking high in risk-aversion, it is even recommendable.
This section continues with the small steps approach and how your long term goals can only be achieved focusing on small deltas every day. This also applies to Information Security programmes and projects.
I like this sentence from the book: "Remember, your next job is probably not your last one".
Lastly, the authors remind you that you are the ultimate control point of both your job (and eventually, your life).
Happy future reading!
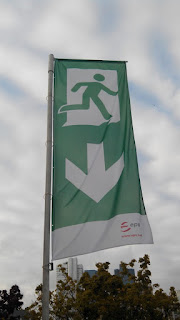 |
Away from monotony! |